The Role of Annotation in Machine Learning and Its Impact on Business
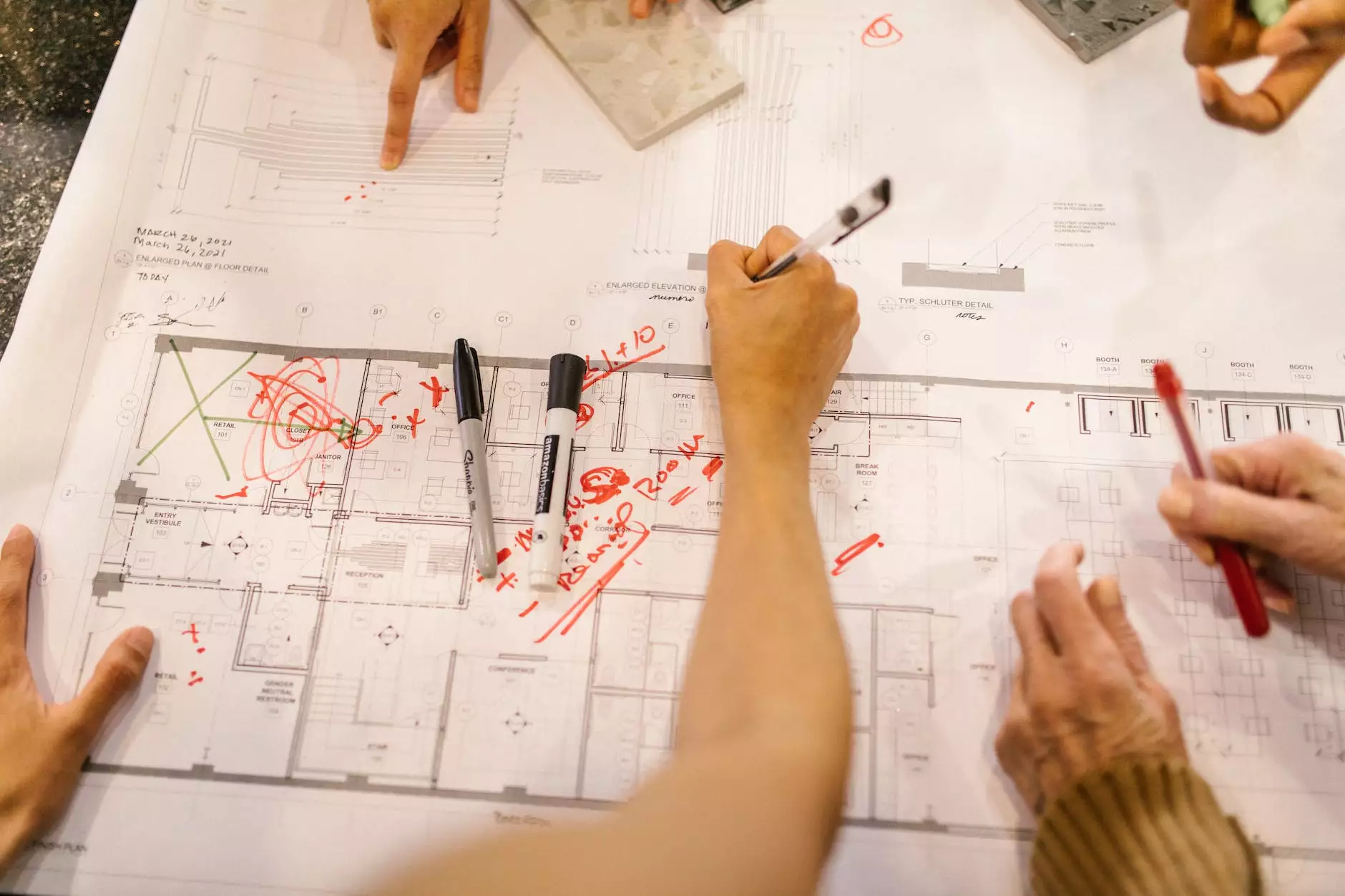
In the fast-evolving digital landscape, businesses are constantly looking for ways to leverage technology to improve operations, streamline services, and enhance customer satisfaction. Among the various technological advancements, annotation in machine learning stands out as a critical element that can drive significant success in sectors such as home services and locksmithing. This article explores how annotation plays a vital role in machine learning and its implications for businesses like KeyMakr.
Understanding Machine Learning and Its Necessity in Business
Machine learning (ML) is a subset of artificial intelligence (AI) that enables systems to learn from data, identify patterns, and make decisions with minimal human intervention. In today's data-driven world, businesses are inundated with vast amounts of information that can be analyzed to derive insights, enhance service delivery, and facilitate customer engagement.
Consider the locksmith industry: from tracking service requests to optimizing inventory based on usage patterns, machine learning can be a game changer. However, the effectiveness of machine learning algorithms heavily relies on the quality of data input. This is where annotation in machine learning comes into play.
What is Annotation in Machine Learning?
Annotation refers to the process of labeling data to provide context that allows machine learning models to learn effectively. This can involve tagging images, transcribing audio, or categorizing text. The primary goal of annotation is to train models so they can perform specific tasks, such as identifying objects in photographs, conducting sentiment analysis on customer reviews, or predicting service demand based on historical data.
Types of Annotation
- Image Annotation: Involves labeling images with relevant tags or landmarks, crucial for computer vision tasks.
- Text Annotation: Includes tasks like named entity recognition and sentiment tagging, which are essential for natural language processing.
- Audio Annotation: Involves transcribing spoken words into text format and labeling audio for better understanding.
The Importance of Quality Annotation for Machine Learning Models
The efficacy of machine learning depends significantly on the quality of data preparation, which includes annotation. Poorly annotated data can lead to inaccurate models, resulting in failures that can affect business outcomes. Here’s how high-quality annotation can impact businesses:
1. Enhanced Decision-Making
With accurate annotations, machine learning models can provide insights that empower businesses to make informed decisions. For example, effective annotation can help a locksmith service accurately forecast demand during peak seasons, ensuring resources are allocated efficiently.
2. Improved Customer Experience
Businesses can utilize machine learning to personalize customer interactions. For instance, a home services company might analyze customer feedback annotated for sentiment to tailor services and address common concerns, leading to enhanced customer satisfaction.
3. Increased Operational Efficiency
By automating tasks that involve data categorization and pattern recognition, businesses can reduce time and costs associated with manual processes. Annotated data feeds machine learning algorithms that optimize scheduling, manage inventory, and streamline operations within various business segments.
Challenges in Annotation
Despite its significance, annotation can be a daunting task for many businesses due to several challenges:
1. Resource-Intensive
Annotating data can require substantial time and human resources, particularly for voluminous datasets common in businesses today.
2. Expertise Required
The complexity of machine learning applications often necessitates skilled annotators who understand both the subject matter and the specific requirements of machine learning tasks.
3. Quality Consistency
Maintaining consistency in annotation across large teams can be difficult, leading to potential errors that adversely affect model performance.
Strategies for Effective Annotation
To overcome these challenges, businesses should adopt the following strategies to ensure their annotation processes are effective and efficient:
1. Utilize Annotating Tools and Software
Leveraging advanced tools can enhance the speed and accuracy of annotation. Options may include image tagging software, transcription systems, and text analysis tools.
2. Train Annotators
Investing in training programs helps ensure that annotators produce high-quality, consistent labels, particularly for nuanced or specialized domains such as locksmith and home services.
3. Implement a Feedback Loop
Establishing a feedback mechanism allows for continuous improvement. Regularly review annotations to identify patterns in errors and make necessary adjustments to training and processes.
Case Study: Annotation in Home Services
Let’s consider a hypothetical example of a home services company utilizing machine learning with superb annotation techniques. By implementing a system to analyze customer requests for locksmith services, the company annotated thousands of service tickets, including details on service type, urgency, location, and customer feedback.
Data Annotation Process
- Collect Data: Gather a significant volume of service requests over a designated period.
- Annotate Data: Label data based on customer reviews, response times, and types of services required.
- Train the Model: Use the annotated dataset to train machine learning models that predict peak service times and customer preferences.
- Evaluate and Adjust: Regularly assess the model’s performance and retrain it with new data for accuracy.
In this case, the application of machine learning, bolstered by clear and effective annotation in machine learning, leads to enhanced decision-making for resource allocation, improved customer satisfaction through personalized services, and ultimately increased revenue for the company.
Conclusion
As businesses like KeyMakr continue to leverage technology for growth and efficiency, the significance of annotation in machine learning cannot be overstated. By ensuring high-quality annotated data, companies can unlock the full potential of their machine learning initiatives, driving not only innovation but also sustained competitive advantage. Whether in the field of locksmith services or broader home service sectors, businesses that prioritize and invest in quality annotation practices will find themselves well-positioned to excel in an increasingly data-driven marketplace.
In summary, the journey towards a highly functional, data-driven business model begins with understanding the crucial role of annotation in machine learning, which underpins successful AI applications across various industries.