The Ultimate Guide to Image Labelling Tools
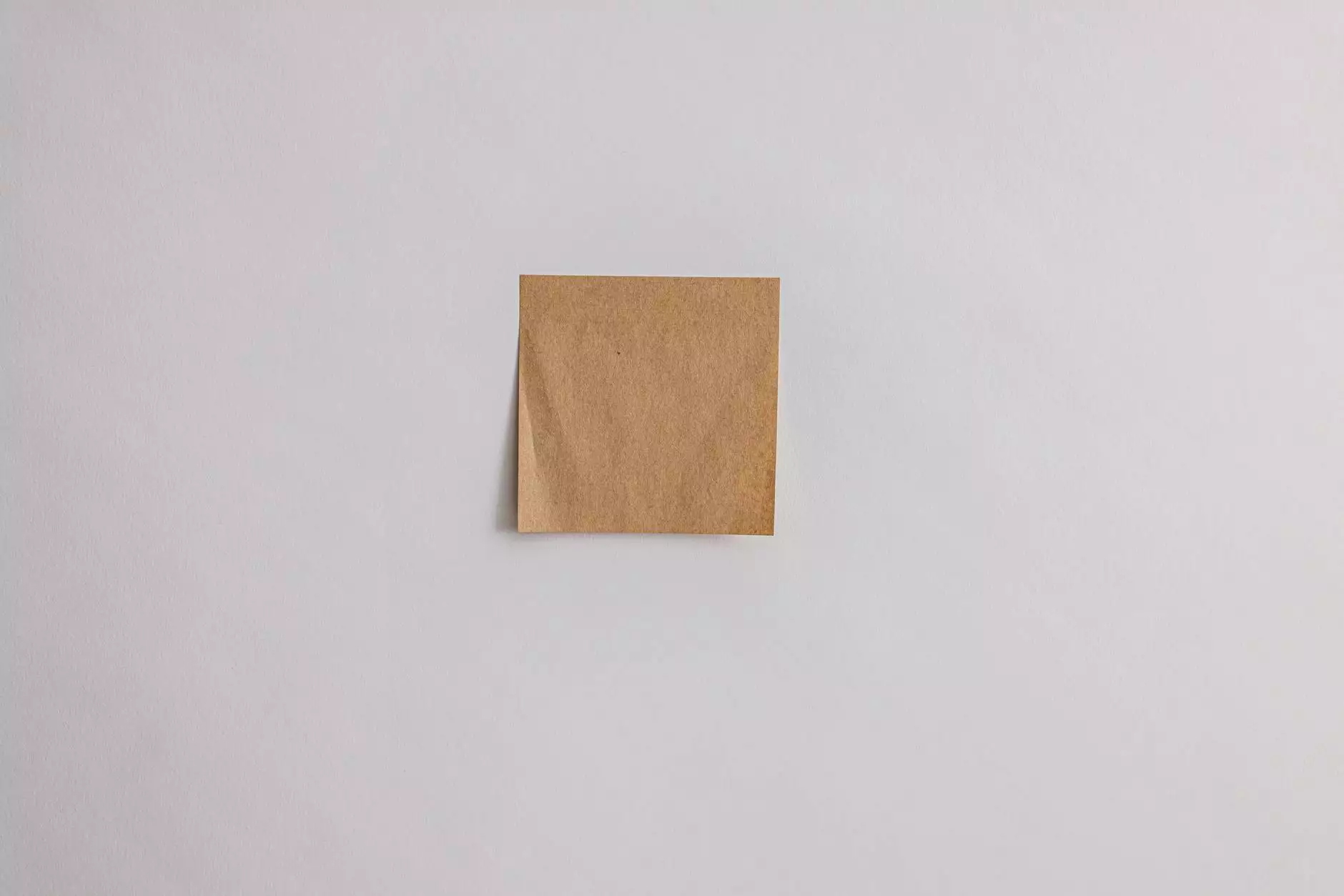
Understanding the Importance of Image Labelling Tools
In today's digital landscape, where data is the new oil, the need for effective data annotation has never been more critical. Image labelling tools allow businesses to efficiently manage and tag visual data, enabling deep learning algorithms to learn effectively. With machine learning and artificial intelligence reshaping various industries, the integration of precise data through image labelling has become an essential step for businesses aiming for success.
What is an Image Labelling Tool?
An image labelling tool is a software solution designed to assist users in tagging images with meaningful annotations. These tools help in categorizing, classifying, and segmenting visual assets. The primary purpose of these tools is to provide detailed labels for images so that AI and machine learning algorithms can understand and learn patterns within the data. Effective annotation reflects on the quality of the models trained on this data, leading to better performance in applications like image recognition, object detection, and more.
Key Features of Image Labelling Tools
Choosing the right image labelling tool can significantly impact your data annotation workflow. Here are some key features to consider:
- User-Friendly Interface: A straightforward interface ensures that your team can start annotating images without significant training.
- Automated Annotation: Some advanced tools offer semi-automated or fully automated image labelling functionalities, saving time and resources.
- Collaboration Tools: Features that enable teams to work together on projects efficiently elevate productivity and accuracy.
- Quality Control: Built-in mechanisms to review and validate annotations, ensuring high-quality data for your projects.
- Integration Capability: The ability to integrate with existing workflows and other software platforms enhances the flexibility of your data pipeline.
- Variety of Annotation Types: Support for various annotation types, including bounding boxes, polygons, and segmentation masks, tailored to different use cases.
The Benefits of Using Image Labelling Tools
Implementing an image labelling tool can yield numerous benefits for businesses:
1. Enhanced Efficiency
The speed of annotating images is significantly increased with these tools. Automated features and batch processing capabilities allow teams to annotate large volumes of images in a fraction of the time it would take manually.
2. Improved Data Accuracy
With built-in quality control features and collaborative environments, image labelling tools help ensure that annotations are accurate and reliable, leading to superior datasets.
3. Scalability
Organizations can scale their annotation efforts according to project demands without a proportional increase in resources, thanks to cloud-based tools and streamlined workflow processes.
4. Cost-Effectiveness
By enhancing productivity and data quality, businesses can reduce the costs associated with retraining models based on faulty data, making image labelling tools a savvy investment.
How to Choose the Right Image Labelling Tool
Selecting the appropriate tool for your needs requires careful consideration. Here are some vital points to evaluate:
- Define Your Requirements: Assess the types of images you'll be annotating and what specific features you require.
- Evaluate User Experience: Test different tools to find the most user-friendly interface for your team.
- Check for Support and Resources: Look for tools backed by reliable customer support and extensive resources, including tutorials and documentation.
- Consider Pricing Models: Understand the pricing structures—be it a subscription model or pay-per-use—to find a solution that fits your budget.
- Look for Integrations: Ensure the tool can integrate seamlessly with your existing systems to minimize disruption in your workflow.
Top Image Labelling Tools in the Market
There are numerous image labelling tools available today, each with its unique features and benefits. Here are some of the most popular:
1. Keylabs.ai
As a leading data annotation platform, Keylabs.ai offers sophisticated image labelling tools combined with powerful AI capabilities. Its collaborative features and flexibility make it a top choice for companies handling large datasets. The intuitive interface ensures that teams can maximize their productivity while maintaining high-quality standards.
2. Labelbox
Labelbox provides robust tools for managing the entire data lifecycle. From data ingestion to quality control, its comprehensive features help streamline the annotation process for various visual data types.
3. VGG Image Annotator
This free tool is favored by academics and smaller projects due to its simplicity and effectiveness. It supports various annotation types while being easy to set up and use.
4. Supervisely
Supervisely is a full-featured data annotation platform designed for computer vision projects. Offering extensive customization and support for advanced annotations, it's an excellent choice for teams needing flexibility and control.
5. Snorkel
Snorkel is a revolution in how data is labelled, primarily using weak supervision techniques. It enables teams to programmatically create training datasets, reducing manual annotation needs.
Best Practices for Using Image Labelling Tools
To get the most out of your image labelling tool, consider following these best practices:
- Create Comprehensive Guidelines: Document clear guidelines for annotators so that everyone follows a standardized approach.
- Train Your Team: Conduct training sessions on the selected tool to familiarize the team with its features and best practices.
- Implement Quality Checks: Regularly review annotated data to catch errors early and maintain high standards.
- Incorporate Feedback: Use annotations feedback to refine guidelines and improve overall accuracy.
- Optimize Workflows: Continuously assess your annotation process and make adjustments to improve efficiency and output.
Future Trends in Image Labelling Tools
The field of data annotation is continuously evolving, with several trends likely to shape the future of image labelling tools:
1. Increased Automation
As machine learning advances, we expect more image labelling tools to integrate automated annotation capabilities, reducing the dependency on manual work.
2. Enhanced Collaboration Features
The need for remote collaboration is becoming more prevalent. Future tools will likely focus on providing better collaborative features for teams operating in different geographies.
3. AI-assisted Annotation
Integration of AI to assist annotators—suggesting labels or highlighting features—will enhance productivity and speed up the annotation process.
4. Adaptation to New Data Types
As technology advances, there will be a greater need to support new data formats, including 3D images and videos, requiring image labelling tools to evolve accordingly.
5. Security and Compliance
As data privacy regulations become more stringent, image labelling tools will need to incorporate strong security measures to protect sensitive data during the annotation process.
Conclusion
Investing in a high-quality image labelling tool can dramatically improve your organization's data annotation processes, boosting the performance of your AI and machine learning initiatives. By selecting the right tool and following best practices, businesses can harness the power of tagged image data to propel their success in a data-driven world. Whether you’re a small startup or a large enterprise, understanding and implementing the best data annotation strategies will position your business ahead of the competition.