Harnessing Machine Learning Against Malware: The Future of Digital Security
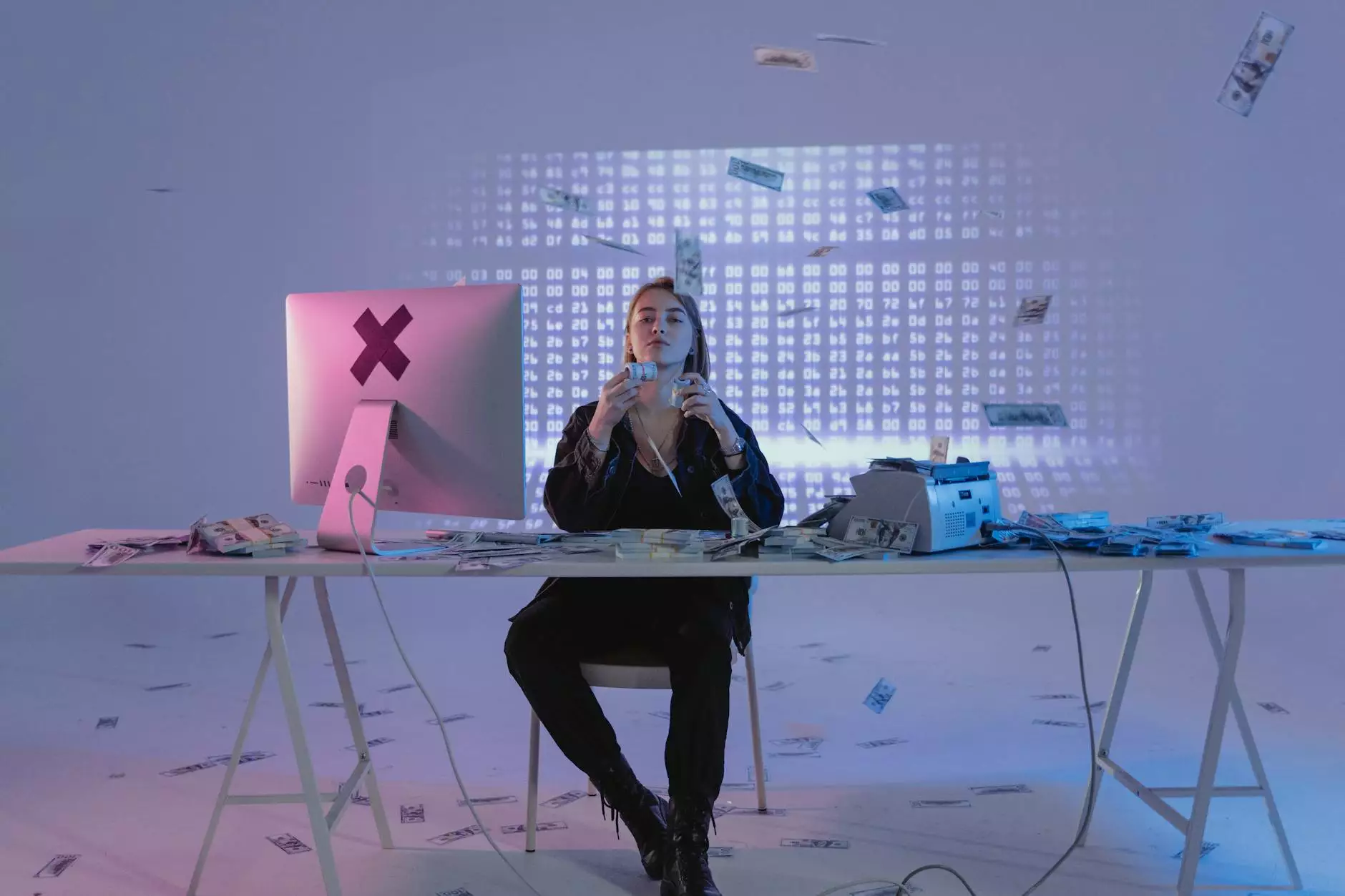
In today's rapidly evolving digital landscape, the threats posed by machine learning malware have become a significant concern for businesses worldwide. With the increasing sophistication of cyber attacks, it is crucial for organizations to adopt innovative solutions that leverage advanced technologies. This article delves into how machine learning can combat malware effectively, benefiting IT services and securing business operations.
Understanding Machine Learning Malware
Machine learning malware refers to malicious software that uses machine learning techniques to enhance its capabilities. Unlike traditional malware, which operates based on predetermined patterns, machine learning malware can learn from its environment. This ability to adapt and evolve poses a substantial threat to cybersecurity.
The Evolution of Malware
To fully grasp the implications of machine learning in malware, it's essential to understand the evolution of malware itself. Over the past few decades, malware has transitioned from simple viruses to complex, multifaceted threats. Key advancements include:
- Polymorphic Malware: This type of malware changes its code as it spreads, making it harder to detect.
- Fileless Malware: Operates in-memory and avoids detection by traditional antivirus solutions that scan for files.
- Ransomware: Locks users out of their files and demands a ransom for access, often employing encryption techniques refined through machine learning.
The Role of Machine Learning in Cybersecurity
Machine learning has emerged as a powerful tool in the fight against malware. By utilizing algorithms that improve automatically through experience, businesses can enhance their security posture in several ways:
1. Enhanced Threat Detection
Machine learning algorithms can analyze vast amounts of data to identify anomalies that may indicate a cyber threat. Traditional security measures often rely on signature-based detection, which falls short against new, unknown malware. Machine learning, however, can detect unusual patterns of behavior, leading to proactive threat hunting and faster incident response.
2. Predictive Analytics
Using historical data, machine learning systems can predict potential future threats. This predictive capability allows organizations to anticipate attacks before they occur, enabling better allocation of resources and timely interventions.
3. Automated Responses
Automation is key to managing modern cyber threats effectively. Machine learning can automate responses to detected threats, minimizing human error and response times. For instance, if a suspicious activity is identified, automatic quarantining or blocking can be triggered, providing immediate protection.
Strategies for Combatting Machine Learning Malware
Organizations need to develop robust strategies that incorporate machine learning principles into their cybersecurity processes:
1. Implementing Advanced Security Solutions
Invest in security solutions that utilize machine learning technologies. These solutions offer more than basic protection; they provide adaptive defenses that evolve as threats change.
2. Continuous Learning and Adaptation
Cybersecurity is not a one-time effort but a continuous process. Organizations should regularly review and update their machine learning models to ensure they are equipped to handle the latest threats. Regular learning from past incidents can enhance the effectiveness of these systems.
3. Training and Awareness
Effective security practices begin with a knowledgeable workforce. Regular training sessions should be conducted to educate employees on the latest threats and the importance of cybersecurity practices.
Real-World Applications of Machine Learning in Malware Defense
Many organizations have begun incorporating machine learning into their cybersecurity frameworks with remarkable success:
1. Amazon Web Services (AWS) GuardDuty
AWS GuardDuty uses machine learning to analyze billions of events across an organization’s AWS accounts, identifying suspicious activity and potential threats in real-time.
2. Google Chronicle
Google Chronicle employs machine learning algorithms to derive insights from security data, providing businesses with the ability to detect, investigate, and respond to threats more efficiently.
Challenges and Considerations
While the integration of machine learning into cybersecurity provides significant benefits, organizations must also be aware of potential challenges:
1. Data Privacy Concerns
With the volume of data being analyzed, there are inherent privacy concerns. Organizations must balance the need for data to train their machine learning models with the necessity of maintaining user privacy.
2. False Positives
Machine learning systems may sometimes flag legitimate activity as a threat, leading to alert fatigue. Organizations must fine-tune their algorithms and continuously improve their models to minimize false positives.
Conclusion: The Future of Cybersecurity with Machine Learning
As machine learning malware continues to evolve, so too must the defenses against it. Organizations that leverage the power of machine learning in their cybersecurity frameworks will not only enhance their threat detection and response capabilities but will also position themselves for a more resilient future.
Investing in advanced IT services, such as those offered by Spambrella, will be paramount in navigating the complexities of modern digital attacks. By understanding the landscape of machine learning in cybersecurity, businesses can forge a more secure path in an increasingly threatening digital world.